|
作者 | Zhu, Yousong1,2 ; Wang, Jinqiao1,2 ; Zhao, Chaoyang1,2 ; Guo, Haiyun1,2 ; Lu, Hanqing1,2
|
出版日期 | 2016
|
会议日期 | 2016.11.20-11.24
|
会议地点 | Taipei, China
|
英文摘要 | Although the Region-based Convolutional Neural Network
(R-CNN) families have shown promising results for object detection,
they still face great challenges for task-specific detection, e.g., pedestrian
detection, the current difficulties of which mainly lie in the large scale
variations of pedestrians and insufficient discriminative power of pedestrian features. To overcome these difficulties, we propose a novel ScaleAdaptive Deconvolutional Regression (SADR) network in this paper.
Specifically, the proposed network can effectively detect pedestrians
of various scales by flexibly choosing which feature layer to regress
object locations according to the height of pedestrians, thus improving
the detection accuracy significantly. Furthermore, considering CNN can
abstract different semantic-level features from different layers, we fuse
features from multiple layers to provide both local characteristics and
global semantic information of the object for final pedestrian classification, which improves the discriminative power of pedestrian features and boosts the detection performance further. Extensive experiments have verified the effectiveness of our proposed approach, which achieves the state-of-the-art log-average miss rate (MR) of 6.94% on the revised Caltech and a competitive result on KITTI. |
源URL | [http://ir.ia.ac.cn/handle/173211/20146] 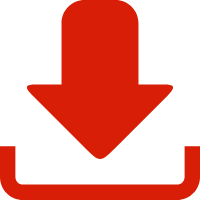 |
专题 | 自动化研究所_模式识别国家重点实验室_图像与视频分析团队
|
作者单位 | 1.Institute of Automation, Chinese Academy of Sciences 2.University of Chinese Academy of Sciences
|
推荐引用方式 GB/T 7714 |
Zhu, Yousong,Wang, Jinqiao,Zhao, Chaoyang,et al. Scale-adaptive Deconvolutional Regression Network for Pedestrian Detection[C]. 见:. Taipei, China. 2016.11.20-11.24.
|