|
作者 | Guo Peng1,2 ; Hong Ma1 ; Ruizhi Chen1,2 ; Pin Li1 ; Shaolin Xie1 ; Donglin Wang1
|
出版日期 | 2018-06
|
会议日期 | 2018-8
|
会议地点 | 爱尔兰都柏林
|
英文摘要 | In recent researches, binarized neural network
(BNN) has been proposed to address the massive computations
and large memory footprint problem of the convolutional neural
network (CNN). Several works have designed specific BNN
accelerators and showed very promising results. Nevertheless,
only part of the neural network is binarized in their architecture and the benefits of binary operations were not fully
exploited. In this work, we propose the first fully binarized
convolutional neural network accelerator (FBNA) architecture,
in which all convolutional operations are binarized and unified,
even including the first layer and padding. The fully unified
architecture provides more resource, parallelism and scalability
optimization opportunities. Compared with the state-of-the-art
BNN accelerator, our evaluation results show 3.1x performance,
5.4x resource efficiency and 4.9x power efficiency on CIFAR-10.
|
源URL | [http://ir.ia.ac.cn/handle/173211/23876] 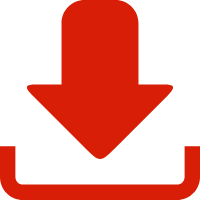 |
专题 | 自动化研究所_国家专用集成电路设计工程技术研究中心
|
通讯作者 | Guo Peng |
作者单位 | 1.中科院自动化所 2.中国科学院大学
|
推荐引用方式 GB/T 7714 |
Guo Peng,Hong Ma,Ruizhi Chen,et al. FBNA: A Fully Binarized Neural Network Accelerator[C]. 见:. 爱尔兰都柏林. 2018-8.
|