|
作者 | Ma, Xuelin1,2 ; Qiu, Shuang1 ; Du, Changde1,2 ; Xing, Jiezhen1,2 ; He, Huiguang1,2,3
|
出版日期 | 2018
|
会议日期 | 18-21 July 2018
|
会议地点 | Honolulu, HI, USA
|
英文摘要 | Motor imagery (MI) based Brain-Computer Interface
(BCI) is an important active BCI paradigm for recognizing
movement intention of severely disabled persons. There are
extensive studies about MI-based intention recognition, most of
which heavily rely on staged handcrafted EEG feature extraction
and classifier design. For end-to-end deep learning methods,
researchers encode spatial information with convolution
neural networks (CNNs) from raw EEG data. Compared with
CNNs, recurrent neural networks (RNNs) allow for long-range
lateral interactions between features. In this paper, we proposed
a pure RNNs-based parallel method for encoding spatial and
temporal sequential raw data with bidirectional Long Short-
Term Memory (bi-LSTM) and standard LSTM, respectively.
Firstly, we rearranged the index of EEG electrodes considering
their spatial location relationship. Secondly, we applied sliding
window method over raw EEG data to obtain more samples
and split them into training and testing sets according to
their original trial index. Thirdly, we utilized the samples and
their transposed matrix as input to the proposed pure RNNsbased
parallel method, which encodes spatial and temporal
information simultaneously. Finally, the proposed method was
evaluated in the public MI-based eegmmidb dataset and compared
with the other three methods (CSP+LDA, FBCSP+LDA,
and CNN-RNN method). The experiment results demonstrated
the superior performance of our proposed pure RNNs-based
parallel method. In the multi-class trial-wise movement intention
classification scenario, our approach obtained an average
accuracy of 68.20% and significantly outperformed other three
methods with an 8.25% improvement of relative accuracy on
average, which proves the feasibility of our approach for the
real-world BCI system. |
源URL | [http://ir.ia.ac.cn/handle/173211/26173] 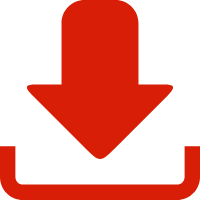 |
专题 | 类脑智能研究中心_神经计算及脑机交互
|
通讯作者 | He, Huiguang |
作者单位 | 1.Research Center for Brain-inspired Intelligence and National Laboratory of Pattern RecognitionInstitute of AutomationChinese Academy of Sciences, Beijing, China 2.University of Chinese Academy of Sciences, Beijing, China 3.Center for Excellence in Brain Science and Intelligence Technology, Chinese Academy of Sciences, Beijing, China
|
推荐引用方式 GB/T 7714 |
Ma, Xuelin,Qiu, Shuang,Du, Changde,et al. Improving EEG-Based Motor Imagery Classification via Spatial and Temporal Recurrent Neural Networks[C]. 见:. Honolulu, HI, USA. 18-21 July 2018.
|