|
作者 | Bingning Wang1,2 ; Ting Yao2; Qi Zhang2; Jingfang Xu2; Zhixing Tian1 ; Kang Liu1 ; Jun Zhao1
|
出版日期 | 2019
|
会议日期 | 2019-7
|
会议地点 | Paris, France
|
英文摘要 | Open-domain question answering focuses on using diverse information resources to answer any types of question. Recent years, with
the development of large-scale data set and various deep neural
networks models, some recent advances in open domain question
answering system first utilize the distantly supervised dataset as
the knowledge resource, then apply deep learning based machine
comprehension techniques to generate the right answers, which
achieves impressive results compared with traditional feature-based
pipeline methods.
However, these deep learning based methods suffer from the inferior quality of the distantly supervised data, and the answer score
is un-normalized among multiple documents. Furthermore, unlike
previous open-domain question answering system, they process
each document independently which may ignore the valuable information in the context. In this paper, we propose a document gated
reader to generate the right answer from multiple documents. We
propose a document-level gate operation to determine the question document relevance and embed it into the answer generation process, and optimize it with the global normalization objective. We
also develop a bootstrapping scheme to obtain high-quality training
data. Experimental results on several question answering datasets
show the advantage of the proposed methods.
|
源URL | [http://ir.ia.ac.cn/handle/173211/44825] 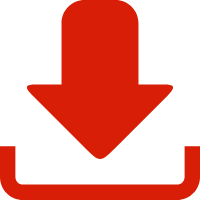 |
专题 | 模式识别国家重点实验室_自然语言处理
|
作者单位 | 1.National Laboratory of Pattern Recognition, Institute of Automation, Chinese Academy of Sciences 2.Sogou Inc.
|
推荐引用方式 GB/T 7714 |
Bingning Wang,Ting Yao,Qi Zhang,et al. Document Gated Reader for Open-Domain Qestion Answering[C]. 见:. Paris, France. 2019-7.
|