|
作者 | Yuanheng Zhu ; Weifan Li ; Mengchen Zhao; Jianye Hao; Dongbin Zhao
|
刊名 | IEEE Transactions on Cybernetics
 |
出版日期 | 2022
|
页码 | doi={10.1109/TCYB.2022.3179775} |
英文摘要 | In single-agent Markov decision processes, an agent
can optimize its policy based on the interaction with the environment.
In multiplayer Markov games (MGs), however, the
interaction is nonstationary due to the behaviors of other players,
so the agent has no fixed optimization objective. The challenge
becomes finding equilibrium policies for all players. In this
research, we treat the evolution of player policies as a dynamical
process and propose a novel learning scheme for Nash equilibrium.
The core is to evolve one’s policy according to not
just its current in-game performance, but an aggregation of its
performance over history. We show that for a variety of MGs,
players in our learning scheme will provably converge to a point
that is an approximation to Nash equilibrium. Combined with
neural networks, we develop an empirical policy optimization
algorithm, which is implemented in a reinforcement-learning
framework and runs in a distributed way, with each player
optimizing its policy based on own observations. We use two
numerical examples to validate the convergence property on
small-scale MGs, and a pong example to show the potential on
large games. |
源URL | [http://ir.ia.ac.cn/handle/173211/51532] 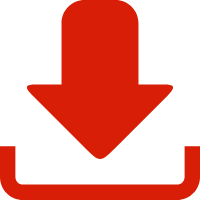 |
专题 | 复杂系统管理与控制国家重点实验室_深度强化学习
|
推荐引用方式 GB/T 7714 |
Yuanheng Zhu,Weifan Li,Mengchen Zhao,et al. Empirical Policy Optimization for n-Player Markov Games[J]. IEEE Transactions on Cybernetics,2022:doi={10.1109/TCYB.2022.3179775}.
|
APA |
Yuanheng Zhu,Weifan Li,Mengchen Zhao,Jianye Hao,&Dongbin Zhao.(2022).Empirical Policy Optimization for n-Player Markov Games.IEEE Transactions on Cybernetics,doi={10.1109/TCYB.2022.3179775}.
|
MLA |
Yuanheng Zhu,et al."Empirical Policy Optimization for n-Player Markov Games".IEEE Transactions on Cybernetics (2022):doi={10.1109/TCYB.2022.3179775}.
|