|
作者 | He Ziwen1,2 ; Wei Wang2 ; Jing Dong2 ; Tieniu Tan2
|
出版日期 | 2022
|
会议日期 | June 21 - 24 2022
|
会议地点 | New Orleans, Louisiana
|
英文摘要 | Deep neural networks have shown their vulnerability to
adversarial attacks. In this paper, we focus on sparse ad-
versarial attack based on the ℓ 0 norm constraint, which can
succeed by only modifying a few pixels of an image. De-
spite a high attack success rate, prior sparse attack meth-
ods achieve a low transferability under the black-box proto-
col due to overfitting the target model. Therefore, we intro-
duce a generator architecture to alleviate the overfitting is-
sue and thus efficiently craft transferable sparse adversarial
examples. Specifically, the generator decouples the sparse
perturbation into amplitude and position components. We
carefullydesignarandomquantizationoperatortooptimize
these two components jointly in an end-to-end way. The
experiment shows that our method has improved the trans-
ferability by a large margin under a similar sparsity set-
ting compared with state-of-the-art methods. Moreover, our
method achieves superior inference speed, 700× faster than
other optimization-based methods. The code is available at
https://github.com/shaguopohuaizhe/TSAA. |
语种 | 英语
|
源URL | [http://ir.ia.ac.cn/handle/173211/51541] 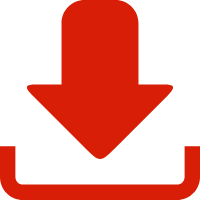 |
专题 | 自动化研究所_智能感知与计算研究中心
|
通讯作者 | Wei Wang |
作者单位 | 1.School of Artificial Intelligence, University of Chinese Academy of Sciences 2.Center for Research on Intelligent Perception and Computing, NLPR, CASIA
|
推荐引用方式 GB/T 7714 |
He Ziwen,Wei Wang,Jing Dong,et al. Transferable sparse adversarial attack[C]. 见:. New Orleans, Louisiana. June 21 - 24 2022.
|