|
作者 | chenguang li ; hongjun yang ; shiying sun ; long cheng
|
出版日期 | 2021-12
|
会议日期 | 2021-12
|
会议地点 | Bali
|
英文摘要 | Assessment of hand motor function is crucial to stroke
patients. However, the commonly used Fugl-Meyer assessment (FMA)
scale requires 11 hand and wrist movements. To simplify these movements,
this study proposes a hand motion classification framework based
on deep learning to achieve the quantitative taxonomy of hand kinematics.
To the best of our knowledge, this is the first study to use deep learning
for the quantitative taxonomy of the hand kinematics. First, we use
the Long Short-Term Memory (LSTM) neural network to extract deep
features of 20 hand movements (including 11 FMA movements) from
37 healthy subjects, and rank the LSTM neural network output value
(predicted probability) of each sample. The similarity between the movements
obtained by the nonlinear transformation can be used to draw the
confusion matrix. Then the confusion matrix is taken as the category feature
to obtain the clustering dendrogram to show the similarity between
different hand movements intuitively. Next, the 20 hand movements are
divided into four groups by hierarchical clustering. The silhouette coefficient
of the clustering results is 0.81, which is close to the ideal value of
1, indicating the validity of the clustering result. Finally, the clustering
center is calculated to find the corresponding movement as the representative
movement for motor function assessment. As a result, we reduced
the 20 movements to 5 movements, allowing for a faster quantitative
assessment of hand motor function than the FMA scale. This also lays
the foundation of the assessment |
源URL | [http://ir.ia.ac.cn/handle/173211/52114] 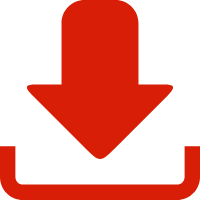 |
专题 | 自动化研究所_复杂系统管理与控制国家重点实验室_先进机器人控制团队
|
通讯作者 | long cheng |
作者单位 | 中国科学院自动化研究所
|
推荐引用方式 GB/T 7714 |
chenguang li,hongjun yang,shiying sun,et al. Quantitative taxonomy of hand kinematics based on long short-term memory neural network[C]. 见:. Bali. 2021-12.
|