|
作者 | Li WF(李伟凡)2 ; Zhu YH(朱圆恒)1,2 ; Zhao DB(赵冬斌)1,2
|
出版日期 | 2023-06
|
会议日期 | 2023-6
|
会议地点 | 昆士兰
|
关键词 | multi-agent
reinforcement learning
policy gradient
|
英文摘要 | We investigate the integration of value-based and
policy gradient methods in multi-agent reinforcement learning
(MARL). The Individual-Global-Max (IGM) principle plays an
important role in value-based MARL, as it ensures consistency
between joint and local action values. IGM is difficult
to guarantee in multi-agent policy gradient methods due to
stochastic exploration and conflicting gradient directions. In
this paper, we propose a novel multi-agent policy gradient
algorithm called Advantage Constrained Proximal Policy Optimization
(ACPPO). ACPPO calculates each agent’s current local
state-action advantage based on their advantage network and
estimates the joint state-action advantage based on multi-agent
advantage decomposition lemma. According to the consistency
of the estimated joint-action advantage and local advantage, the
coefficient of each agent constrains the joint-action advantage.
ACPPO, unlike previous policy gradient MARL algorithms, does
not require an additional sampled baseline to reduce variance
or a sequential scheme to improve accuracy. The proposed
method is evaluated using the continuous matrix game, the
Starcraft Multi-Agent Challenge, and the Multi-Agent MuJoCo
task. ACPPO outperforms baselines such as MAPPO, MADDPG,
and HATRPO, according to the results. |
源URL | [http://ir.ia.ac.cn/handle/173211/52273] 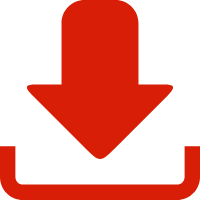 |
专题 | 复杂系统管理与控制国家重点实验室_深度强化学习
|
通讯作者 | Zhu YH(朱圆恒) |
作者单位 | 1.School of artificial intelligence, University of Chinese Academy of Sciences, Beijing 101408, China 2.State Key Laboratory of Multimodal Artificial Intelligence Systems, Institute of Automation, Chinese Academy of Sciences, Beijing 100190, China
|
推荐引用方式 GB/T 7714 |
Li WF,Zhu YH,Zhao DB. Advantage Constrained Proximal Policy Optimization in Multi-Agent Reinforcement Learning[C]. 见:. 昆士兰. 2023-6.
|