|
作者 | Xu BW(许宝文)1,2 ; Wang XL(王学雷)2 ; Liu ZJ(刘振杰)2 ; Kang LW(康丽雯)1,2
|
出版日期 | 2023-09
|
会议日期 | 2023-11
|
会议地点 | New York
|
英文摘要 | Traffic prediction is an important component of intelligent transportation
systems. However, due to the dynamic and complex dependence
of time and space, traffic prediction is extremely challenging.
In terms of time correlation, due to the impact of external
events such as traffic accidents and weather conditions, there are
non-stationary signals in traffic sequence signals. This means that
future data cannot be inferred only from past periodicity, and its
state may suddenly change at any moment. The existing methods
still have two limitations. Firstly, when modeling spatial dependencies,
many methods only build graphs that contain a small portion
of task information, limiting the expressive power of the model. Secondly,
when modeling temporal correlation, many methods cannot
effectively solve the problem of non-stationary signal prediction
in traffic data signals. Therefore, we propose a GAN (GCGAN)
that combines graphic contrast learning. Specifically, in the generator,
we construct a graph containing as much prediction task
information as possible through graph comparison learning, and
calculate the similarity between two time series by using Dynamic
Time Warping (DTW) instead of Euclidean distance, thereby accurately
predicting mutations. Then we design a discriminator to
maintain global consistency between the predicted time series and
the ground reality. This method can effectively solve the limitations
of existing methods and improve the accuracy and reliability of
traffic prediction. |
语种 | 英语
|
源URL | [http://ir.ia.ac.cn/handle/173211/57606] 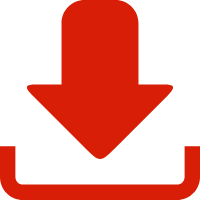 |
专题 | 综合信息系统研究中心_工业智能技术与系统
|
通讯作者 | Wang XL(王学雷) |
作者单位 | 1.中国科学院大学人工智能学院 2.中国科学院自动化研究所
|
推荐引用方式 GB/T 7714 |
Xu BW,Wang XL,Liu ZJ,et al. A GAN combined with Graph Contrastive Learning for Traffic Forecasting[C]. 见:. New York. 2023-11.
|