|
作者 | Xu BW(许宝文)1,2 ; Wang XL(王学雷)2 ; Li S(李铄)1,2; Li JW(李经纬)1,2 ; Liu CB(刘承宝)2
|
出版日期 | 2023-09
|
会议日期 | 2023-11
|
会议地点 | changshao, china
|
英文摘要 | Pedestrian trajectory prediction is a fundamental task in applications
such as autonomous driving, robot navigation, and advanced
video surveillance. Since human motion behavior is inherently unpredictable,
resembling a process of decision-making and intrinsic motivation,
it naturally exhibits multimodality and uncertainty. Therefore,
predicting multi-modal future trajectories in a reasonable manner poses
challenges. The goal of multi-modal pedestrian trajectory prediction is to
forecast multiple socially plausible future motion paths based on the historical
motion paths of agents. In this paper, we propose a multi-modal
pedestrian trajectory prediction method based on conditional variational
auto-encoder. Specifically, the core of the proposed model is a conditional
variational auto-encoder architecture that learns the distribution
of future trajectories of agents by leveraging random latent variables
conditioned on observed past trajectories. The encoder models the channel
and temporal dimensions of historical agent trajectories sequentially,
incorporating channel attention and self-attention to dynamically extract
spatio-temporal features of observed past trajectories. The decoder
is bidirectional, first estimating the future trajectory endpoints of the
agents and then using the estimated trajectory endpoints as the starting
position for the backward decoder to predict future trajectories from
both directions, reducing cumulative errors over longer prediction ranges.
The proposed model is evaluated on the widely used ETH/UCY pedestrian
trajectory prediction benchmark and achieves state-of-the-art performance. |
源URL | [http://ir.ia.ac.cn/handle/173211/57611] 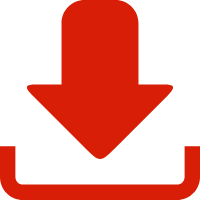 |
专题 | 综合信息系统研究中心_工业智能技术与系统
|
作者单位 | 1.中国科学院大学人工智能学院 2.中国科学院自动化研究所
|
推荐引用方式 GB/T 7714 |
Xu BW,Wang XL,Li S,et al. Social-CVAE: Pedestrian Trajectory Prediction using Conditional Variational Auto-Encoder[C]. 见:. changshao, china. 2023-11.
|